The potential of AI and generative AI (GenAI) to revolutionize investment management is undeniable. The real question isn't if these technologies will be adopted—it's how firms will successfully integrate them at scale.
GenAI Proofs of Concept (PoCs) in investment management offer valuable insights and demonstrate alignment with strategic objectives. Yet, the true test lies in transitioning from these initial experiments to sustainable, scalable operations. In this blog, we dive into the challenges investment managers must overcome and the key strategies needed to turn the latest AI innovations into long-term success.
Learn more about how Linedata partners with clients to scale AI solutions within their operations.
Navigating AI PoCs: Common Pitfalls and Success Strategies
Recent advances in AI, especially GenAI, have highlighted its transformative potential in financial services, from fraud detection and risk management to customer service and investment operations. AI's ability to analyze vast amounts of data at unprecedented speeds enhances decision-making, uncovers hidden patterns, and generates previously unattainable insights.
To explore these possibilities, firms conduct Proofs of Concept (PoCs )which quickly test hypotheses with limited scope and cost before committing to full-scale implementation. Typically, a small team of stakeholders, a data scientist, and someone with data access are involved. The primary goal is to identify use cases with the highest benefit/cost ratio and secure executive buy-in for further development.
However, many organizations struggle to transition from AI PoCs to full-scale production. Successfully operationalizing AI and understanding why many initiatives fail is crucial for leveraging AI to drive innovation in financial services rather than falling short of its potential.
The Roadmap from Pilot to Production
Market research indicates a steady increase in the absolute number of AI projects that reach production. But the story is mixed. Today, there is much more active experimentation at all stages of GenAi in investment management and other AI projects than prior to November 2022, when ChatGPT and GenAI burst on the scene.
Yet, Gartner reports that up to 30% of such projects fail to deliver on their promise and are abandoned after less than a year. Bain points to a lack of operating model alignment as one reason. Both statistics highlight the substantial gap between AI’s potential and its practical outcomes.
Organizational requirements beyond technology
Successfully transitioning AI from pilot to production requires more than technical expertise; it demands a holistic strategy integrating technology, data management, AI model development, and governance.
Crucially, it also involves fostering organizational change.
As PoC workflows evolve, organizations must prioritize effective communication, robust onboarding, and continuous training to ensure all stakeholders adapt smoothly to new systems and practices.
Before we delve into the technical aspects of ensuring an efficient move to production, it is vital to properly define the success criteria from the outset.
- What business problem does the PoC address?
- Are there measurable metrics?
- How does the use case align with our business strategy?
- Does the project include a plan for organizational change?
LinkedIn Poll highlights data challenges in AI PoC deployment
Linedata's recent poll identified data integration challenges as the most significant hurdle facing financial services firms in realizing business benefits from their AI PoCs
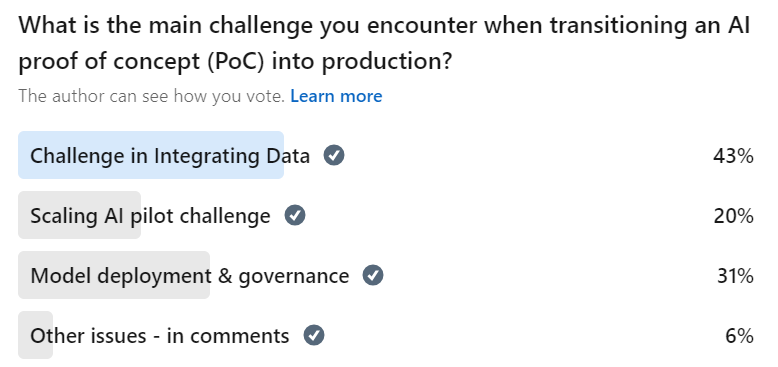
Scaling PoC Data Requirements
AI models rely heavily on data. In the PoC phase, data requirements may be manageable, but scaling AI in investment management to production can reveal new challenges.
Data Quality and Integrity: Firms often use a mix of proprietary and third-party applications for various workflows, each with its own data model. Mapping data points to a common model and ensuring daily reliability and accuracy is challenging. Data quality issues can lead to unreliable AI outputs.
Data Volume and Velocity: Production environments handle larger data volumes at higher velocities, unlike PoCs, which use a static subset of data. Appropriate infrastructure must be deployed to scale into production to meet these demands.
Data Selection: A common mistake during pilots is choosing data that doesn't represent actual production data. Synthesized or narrow data can yield great initial results but fail in real-life scenarios. Proper data selection is crucial for ensuring PoC models remain relevant in production.
Data Governance: Sensitive data must be masked to prevent bias in the model. While this is often done manually during PoC model training, it should be integrated into data ingestion pipelines to avoid leakage. Robust data governance practices are crucial for AI implementation, ensuring compliance with regulations like GDPR or CCPA, especially in the highly regulated financial sector.
Ensuring Robust Infrastructure and Integration
AI solutions must integrate with existing systems and infrastructure for financial firms to derive real value. Key considerations include:
Scalability: PoC scaling needs infrastructure to handle increasing loads without degradation. Therefore, AI solutions are often deployed on public or private clouds for flexibility.
Integration: Ideally, an AI application would access all necessary data from a single, clean source, but this is rarely the case. To be practical, model outputs must be integrated with existing applications, so ensuring smooth interactions through custom APIs or middleware is crucial.
Dev Ops Capabilities Like any technology, AI applications need automation in CI/CD to minimize manual work and prevent human errors. This should be a mandatory part of operationalization.
Monitoring Model Performance and Ongoing Governance
The model that worked well in a PoC may need optimization to perform effectively at scale. AI systems are not "set it and forget it" solutions but require ongoing monitoring and maintenance including:
Model Refinement: Business environments are dynamic, and thus, the AI models that worked six months ago may not be applicable in the current environment. Proper monitoring tools, including feedback loops, are critical to tracking performance and alerting about deviations.
ML Ops / Maintenance Routines: Like traditional Dev Ops, ML Ops capabilities ensure seamless model deployments when new versions are available. Maintenance routines must be developed and followed to ensure that models remain effective and that infrastructure underpins AI operations.
How Linedata Partners with Clients: Scaling AI in Investment Management
Building an AI PoC has been democratized due to the abundance of tools available in the marketplace. Transitioning to Production, however, is challenging, as discussed above. Other issues include a skills shortage, the relative cost-effectiveness of deploying internal resources, and the need for regular maintenance and updates. This is all in addition to setting up dedicated infrastructure and incurring ongoing costs.
At Linedata, we have partnered with many clients on their AI journeys. As they prepare to scale PoCs, our team provides expertise in AI implementation, including Gen AI/ RAG techniques, access to cutting-edge technology, and cost efficiency, enabling faster development and resource flexibility.
Transitioning and scaling AI from PoC to full-scale production in investment management requires careful planning, robust infrastructure, and proactive management. Financial firms can increase the likelihood of successful implementation through organizational change management and by partnering with an expert team to ensure effective data management, optimized model performance, and monitoring.
Learn more
Read about how Linedata is partnering with investment managers to supercharge their operations with these real-world generative AI use cases or contact Ashmita to start a conversation or see a demo.
About the author, Ashmita Gupta
Ashmita Gupta is a SVP and Chartered Financial Analyst (CFA) who heads Linedata’s AI and Analytics. She is passionate about helping clients solve operational problems and open up their teams to innovation, tapping into their data with AI/ML insights.